Neuromorphic Computing: The Future of AI That Thinks Like a Human
Neuromorphic computing is the future of AI, mimicking the human brain for faster, energy-efficient, and adaptive machine learning. Discover its impact on AI and beyond.
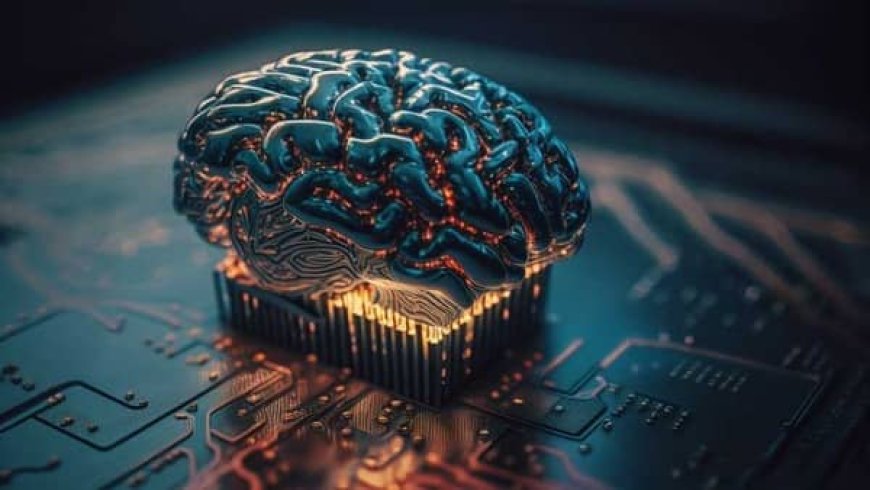
Introduction
What if computers could think like humans, process information like our brains, and learn in real-time without relying on traditional programming? Enter neuromorphic computing—a revolutionary approach to artificial intelligence (AI) that mimics the human brain’s neural networks to create smarter, faster, and more efficient machines.
Unlike conventional computers, which process data sequentially, neuromorphic systems use brain-inspired architectures to process information in parallel, enabling lightning-fast decision-making, ultra-low power consumption, and real-time learning. Tech giants like Intel, IBM, and Qualcomm are already developing neuromorphic chips that could redefine AI, robotics, and even neuroscience.
So, how does neuromorphic computing work, and why is it considered the future of AI? Let’s dive in.
What Is Neuromorphic Computing?
Neuromorphic computing is a field of AI that replicates the structure and functionality of the human brain to enhance machine intelligence. The term was first coined in the 1980s by Carver Mead, a pioneer in silicon-based neural networks.
Traditional computers rely on Von Neumann architecture, where data is processed in a linear fashion, moving between the CPU and memory. In contrast, neuromorphic systems use spiking neural networks (SNNs)—inspired by biological neurons—to process information simultaneously, just like the brain.
This brain-like computing approach enables machines to:
✅ Process information faster than traditional AI models
✅ Consume significantly less energy compared to classical supercomputers
✅ Adapt and learn in real-time without massive datasets
✅ Handle complex pattern recognition tasks more efficiently
How Does Neuromorphic Computing Work?
Neuromorphic computing is built on three core principles that mirror biological brains:
1. Artificial Neurons & Synapses
Instead of traditional transistors, neuromorphic chips use artificial neurons and synapses that communicate through electrical spikes—just like real neurons. These artificial synapses adjust their strength dynamically, enabling adaptive learning and parallel processing.
2. Spiking Neural Networks (SNNs)
Unlike deep learning models that require extensive training with labeled data, SNNs process data asynchronously, meaning they only activate when needed. This reduces power consumption and allows for real-time processing of sensory inputs, such as vision and speech.
3. Event-Driven Processing
Neuromorphic systems are event-driven, meaning they respond to stimuli just like the human brain. This allows them to efficiently handle dynamic environments, making them ideal for robotics, autonomous vehicles, and real-time decision-making AI.
Why Neuromorphic Computing Is a Game-Changer
Neuromorphic computing has the potential to overcome the limitations of traditional AI and deep learning. Here’s why it’s a game-changer:
* Ultra-Efficient AI Processing
Modern AI models require massive amounts of energy to process data. Neuromorphic chips, on the other hand, consume 1000x less power by mimicking how neurons communicate, making AI more sustainable.
* Real-Time Learning & Adaptability
Unlike deep learning models that need constant retraining, neuromorphic systems adapt on the fly, just like human brains. This makes them perfect for applications like edge computing, autonomous robots, and real-time AI assistants.
* Better AI for Healthcare & Neuroscience
Since neuromorphic chips function like biological brains, they can simulate neurological disorders, helping researchers understand conditions like Alzheimer’s, Parkinson’s, and epilepsy better than ever before.
* Smarter & Safer Autonomous Systems
From self-driving cars to drones and robotics, neuromorphic computing enables machines to react in real-time, improving safety and efficiency. Intel’s Loihi chip, for example, can process sensory data with minimal latency, making autonomous systems more responsive.
Real-World Applications of Neuromorphic Computing
* AI-Powered Edge Devices – Smartphones, wearables, and IoT devices could run powerful AI models with minimal energy consumption.
* Autonomous Vehicles & Drones – Self-driving cars could make split-second decisions without relying on cloud computing, reducing lag and improving safety.
* Medical Diagnostics & Brain Research – Simulating brain functions could help in early disease detection, prosthetics, and personalized treatments.
* Smart Surveillance & Security – Low-power AI cameras could detect threats in real-time without consuming excessive bandwidth.
* Space Exploration & Robotics – NASA is exploring neuromorphic chips for AI-driven space missions, where power efficiency is crucial.
Challenges in Neuromorphic Computing
While neuromorphic computing is incredibly promising, several challenges remain before it becomes mainstream:
* Hardware Development – Current silicon-based chips are not optimized for neuromorphic architectures. Researchers are exploring memristors and analog computing to bridge this gap.
* Lack of Standardization – Unlike classical computing, neuromorphic systems do not yet have standardized programming models. Companies are developing new AI frameworks tailored for brain-like processing.
* Scalability Issues – While neuromorphic chips excel at small-scale tasks, scaling them to handle large datasets and complex AI models is still a challenge.
* Integration with Existing AI Models – Most AI systems today are designed for deep learning. Adapting them for neuromorphic architectures requires a complete rethink of AI algorithms.
However, with companies like IBM (TrueNorth), Intel (Loihi), and BrainChip (Akida) leading the charge, breakthroughs are happening at an accelerated pace.
The Future of Neuromorphic Computing
Experts predict that neuromorphic AI will become mainstream within the next 10 to 15 years, transforming industries from healthcare to robotics. In the future, we could see:
✔️ AI-powered neuromorphic processors in everyday devices
✔️ Brain-machine interfaces that allow direct communication between humans and computers
✔️ Energy-efficient supercomputers solving complex scientific problems
✔️ Fully autonomous AI assistants that think and learn like humans
As neuromorphic computing advances, it will revolutionize AI, making it smarter, faster, and more human-like than ever before.
Final Thoughts
Neuromorphic computing is more than just the next step in AI—it’s a complete paradigm shift that brings machines closer to human intelligence. With its unmatched efficiency, adaptability, and brain-inspired processing, this cutting-edge technology could reshape the future of artificial intelligence as we know it.
As research accelerates, we may soon live in a world where AI doesn’t just follow algorithms—it thinks, learns, and reacts like us. The age of neuromorphic computing is just beginning, and the possibilities are limitless.
What's Your Reaction?
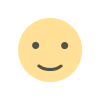
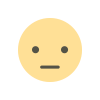
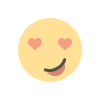
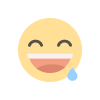
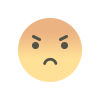
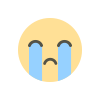
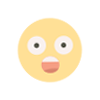